Introduction
Targeted drug therapy has proven to be a highly advantageous approach to cancer treatments, presenting high efficiency and low patient drug resistance.
Yet, there are drawbacks to the use of targeted drug therapy for cancer, including a lack of identified druggable genomic targets that extend across the patient population. Artificial intelligence algorithms can help researchers better understand carcinogenesis and identify new cancer targets.
Artificial Intelligence (AI) is a field combining computer science and extensive data sets to perceive, understand, and solve problems.
Two major branches of AI applied biologically are machine learning-based and network-based. Machine learning is an application of AI wherein a computer can learn without direct instruction through mathematical models and pattern recognition. Network-based AI sorts and compares data, providing and compensating different perspectives.
The Food and Drug Administration (FDA) and the international community have presented an increased interest in trustworthy and ethical AI adoption and innovation.
Applications
The development of multiomics technology is a factor that has bolstered the process of identifying novel anti-cancer targets. Multiomics is an approach to biological analysis that consists of forming data sets out of “omics”, which in the case of cancer are epigenetics, genomics, proteomics, and metabolomics. AI can analyze these data sets to investigate for anti-cancer targets.
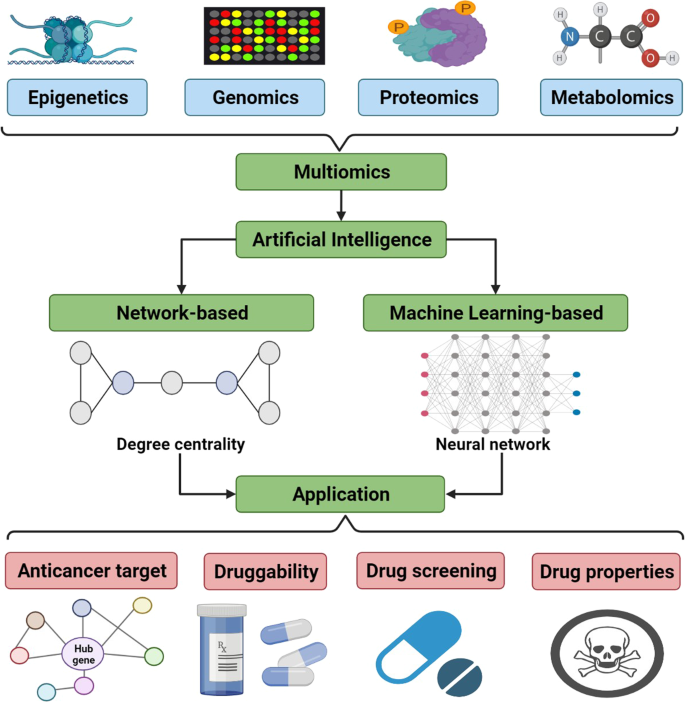
Epigenetics
Unlike the changes in the nucleotide sequence characteristic of genetic mutation, epigenetics is similar to an “on-and-off” switch for the expression of specific genetic attributes without altering the genome. Cancer can be caused by both a genetic mutation or an epigenetic signal gone awry.
A major problem facing epigenetics cancer research is finding specific gene patterns that predict which patients will respond to a cancer treatment. The study of the reversal of epigenetic modifications through AI can give insight into how exactly healthy cells become cancerous and which genetic marker within a patient will respond to cancer-treating drugs.
Genomics
The study of genomics involves the mapping of an entire genome including its structure, function, and evolution, through genome-wide assays such as sequencing. A network-based AI is capable of finding similarities in specific genetic sequences and patterns in their phenotypic expression and interactions. Cancerous biomarkers can be identified through genomic data sets, identifying which genes medical professionals should consider oncogenes of interest.
Proteomics
Proteomics refers to proteins and their interactions within the body. Protein-protein interaction (PPI) research classifies a certain type of protein as “indispensable” and associates them as a major site of disease-causing mutations and drug targeting. In a 2017 study, Ravindran et al. found by analyzing the human PPI data from cancer patients that there are 56 indispensable genes in nine cancers, 46 of which were associated with getting cancer for the first time. This protein interaction data can be harnessed through AI to reveal novel cancer-associated interactions and their potential drug targets.
Metabolomics
Metabolomics is the study of metabolites: the substrates, intermediates, and products of the human metabolic pathway. A hallmark of cancer cells is that they work to alter these metabolites in order to support their rapid growth, providing energy to their biosynthetic pathways and changing their redox balance. Cancer can be detected through biological AI network analysis due to the presence of certain biomarker metabolites within biofluids, cells, and tissues of the body.
Conclusion
All aforementioned “omics” data can be presented for review in the form of multiomics integration analysis. Varied and interconnected data in a network format allows researchers to study carcinogenesis and drug targeting from an overarching perspective in a multifaceted group of patients.
While treatment of cancer remains a formidable challenge, rapid technological advances in data collection and analysis through the use of artificial intelligence combined with robust information exchange may prove to be increasingly beneficial to the way cancer drugs are created and tested, leading to potential a safer and healthier world.
References
- Breakthroughs Staff. (2017, December 12). Treating Cancer by Using Epigenetics, the ‘Software’ of Our Genes | Pfizer. Pfizer News. https://www.pfizer.com/news/articles/treating-cancer-using-epigenetics-%E2%80%98software%E2%80%99-our-genes
- Guenthoer, J., Lilly, M., Starr, T. N., Dadonaite, B., Lovendahl, K. N., Croft, J. T., Stoddard, C. I., Chohan, V., Ding, S., Ruiz, F., Kopp, M. S., Finzi, A., Bloom, J. D., Chu, H. Y., Lee, K. K., & Overbaugh, J. (2023). Identification of broad, potent antibodies to functionally constrained regions of SARS-CoV-2 spike following a breakthrough infection. Proceedings of the National Academy of Sciences, 120(23), e2220948120. https://doi.org/10.1073/pnas.2220948120
- Huang, S., Wang, Z., & Zhao, L. (2021). The Crucial Roles of Intermediate Metabolites in Cancer. Cancer Management and Research, 13, 6291–6307. https://doi.org/10.2147/CMAR.S321433
- Ravindran, V., V., S., & Bagler, G. (2017). Identification of critical regulatory genes in cancer signaling network using controllability analysis. Physica A: Statistical Mechanics and Its Applications, 474, 134–143. https://doi.org/10.1016/j.physa.2017.01.059
- U.S. Food and Drug Administration. (2022). Using Artificial Intelligence & Machine Learning in the Development of Drug & Biological Products. https://www.fda.gov/media/167973/download
- You, Y., Lai, X., Pan, Y., Zheng, H., Vera, J., Liu, S., Deng, S., & Zhang, L. (2022). Artificial intelligence in cancer target identification and drug discovery. Signal Transduction and Targeted Therapy, 7(1), Article 1. https://doi.org/10.1038/s41392-022-00994-0
Jordan is a Chemical Engineering student and undergraduate researcher at the University of Florida. She is passionate about genetic engineering and science communication. Her goal is to portray scientific information in a way that is understandable and beneficial to everyone, whether they are immersed in the scientific community or not.